In the previous post, I examined the affects of warnings with more area on a single isolated long-tracked tornadic storm. Now let’s look at a typical event that can comprise multiple tornadoes from independent mesocyclonic circulations, namely tornadoes along the leading edge of a Quasi-Linear Convective System (QCLS). These are typically more challenging than supercell tornadoes to warn for because their existence, timing, and location can be more uncertain. An example QLCS system with 5 vortices along the leading edge of the gust front is depicted here:
How are these situations warned for today? Well, the strategies vary from WFO to WFO and from warning forecaster to warning forecaster. In some instances, the large uncertainty of the event and sometimes high workload encourage forecasters to “cast a wide net” over the entire leading edge of the QLCS system with a single large and long Tornado Warning in hopes of not missing any tornado that might occur. However, this may result and large areas falsely warned for considerable amounts of time. But if there are no tornadoes, it’s only one false alarm. One of the pitfalls of our current warning verification system!
In other instances, forecasters might be more conservative and wait until a signature becomes apparent, and issue a smaller more-precise Tornado Warning for just that particular circulation. However, in these instances, sometimes the warnings come late, resulting in either small lead times, or negative lead times with some or all of the portions of the tornadoes being missed.
Let’s look at how both warning methodologies stack up when verified geospatially for a simulated QLCS event. For the simulation, I created 5 parallel mesocyclone tracks that were 40 km apart, each starting and ending at the same time with a 60 minute duration and moving with the same motion vector (about 25 m/s from the southwest to the northeast). As with the Tuscaloosa case, I used the mesocyclone centroid locations to determine the storm motion vector and position of the warning polygons, simulating a storm motion forecast with perfect skill using careful radar analysis.
I ran two warning methodologies. In each case, the warnings expire after their intended duration and are never modified using the Severe Weather Statement (SVS) reduction method during their durations. The warnings start at the same time in each scenario.
1warn: Issue one large warning using the default AWIPS WarnGen “line warning” polygon with an expiration time of 60 minutes. To create the warning, a line is drawn from the centroid of the first mesocyclone to the centroid of the fifth mesocyclone at the beginning time of the warning. This line is projected ahead 60 minutes using the storm motion vector. A 10 km buffer is drawn surrounding the beginning line and a 15 km buffer is drawn around the ending line. These are the same parameters used to create the default AWIPS WarnGen polygons for line warnings. The far corners of each buffer are connected to create a four-sided polygon. This is the “cast the wide net” scenario.
5warn: Issue five small warnings centered on each of the five individual mesocyclones. The warning durations are set for 30 minutes (half the duration). The warning polygon sizes are based on a 5 km buffer (10 km back edge) around the starting point of each warning, and a 10 km buffer (20 km front edge) around the projected ending point of each warning. Note that these are smaller than the default buffer sizes, and are chosen so to simulate a more precise warning for what are typically short-lived tornadoes. This is the “high precision” scenario.
I then vary the outcome, the verification of the tornado events, in two ways:
1torn: Only one tornado: The middle (third) mesocyclone produces a tornado 10 minutes after the warnings were issued, lasting exactly 10 minutes. The other four mesocyclones are non-tornadic.
5torn: Five tornadoes: All five mesocyclones produce a tornado 10 minutes after the warnings were issued, each lasting exactly 10 minutes.
The four scenarios are illustrated in the figure below. In each case, the dark regions inside the polygons represents the area swept out by the simulated tornadoes with a 5 km splat radius added.
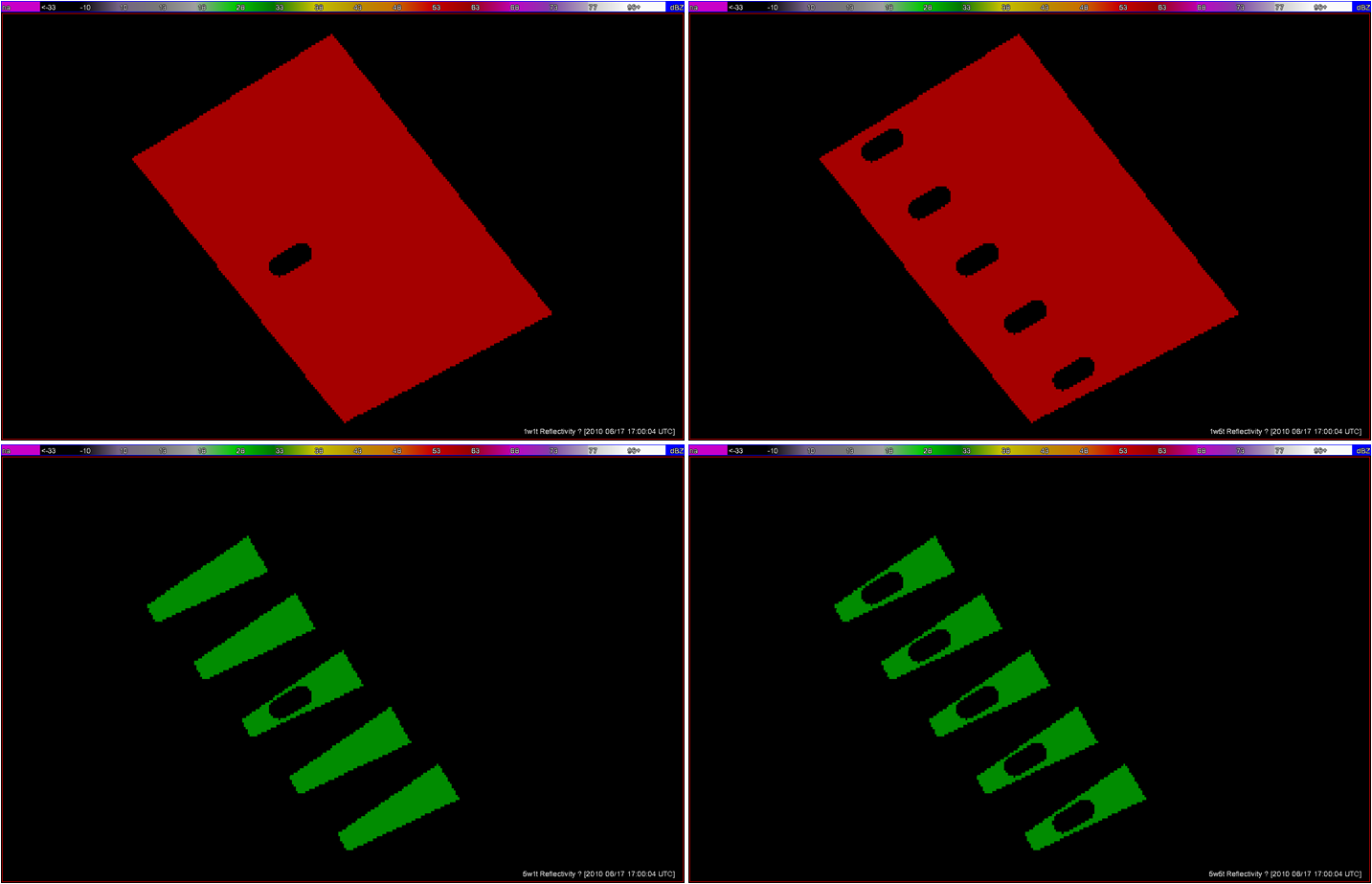
Let’s first consider the traditional warning verification stats for these four scenarios:
1warn-1torn: POD 1.0, FAR 0.0, CSI 1.0
5warn-1torn: POD 1.0, FAR 0.8, CSI 0.2
1warn-5torn: POD 1.0, FAR 0.0, CSI 1.0
5warn-5torn: POD 1.0, FAR 0.0, CSI 1.0
All but one of the scenarios results in prefect warning verification. Scenario 5warn-1torn, 5 precise warnings and only one tornado, results in 4 “false alarms”.
Now, how do these scores compare to using geospatial warning verification, where only one 2×2 table is used, and large false alarm area and times are considered poor performance? Using the “grid point” verification method:
1warn-1torn: POD 1.0000, FAR 0.9993, CSI 0.0007
5warn-1torn: POD 1.0000, FAR 0.9942, CSI 0.0058
1warn-5torn: POD 1.0000, FAR 0.9966, CSI 0.0034
5warn-5torn: POD 1.0000, FAR 0.9709, CSI 0.0291
And using the “truth event” verification method:
1warn-1torn: POD 1.0000, FAR 0.9894, CSI 0.0106
5warn-1torn: POD 1.0000, FAR 0.9544, CSI 0.0456
1warn-5torn: POD 1.0000, FAR 0.9471, CSI 0.0529
5warn-5torn: POD 1.0000, FAR 0.7726, CSI 0.2274
Using each of these verification methods, the scenarios in which 5 separate precision warnings are issued have lower FARs and higher CSIs versus the large long-duration warning decisions for both the 1-tornado event and the 5-tornado events respectively. In addition, there is the obvious difference in the scores when comparing the aggregate false alarm times for each scenario:
1warn-1torn: FAT 1,101,120 km2-sec
5warn-1torn: FAT 123,660 km2-sec
1warn-5torn: FAT 1,054,020 km2-sec
5warn-5torn: FAT 100,110 km2-sec
The 5 precision warnings result in total false alarm times that are about 1/10th of the large long-duration warnings. So even given the case that 5 precision warnings are issued and only one tornado results, the time and area under false alarm is greatly reduced.
From a services standpoint, in the 5 precision warning case, there will be 5 separate alerts, 4 resulting in no tornado. However, for the large warning scenario, there may only be “one alert”, but 10 times the area and time receiving the alert result in no tornado. How do we deal with the issue that there will be more false alerts for the precision warning method? We change the way we alert! More later…
ADDENDUM: Jim LaDue of the NWS Warning Decision Training Branch (WDTB) wrote an excellent position paper describing why we should not adopt a practice to avoid issuing Tornado Warnings (and instead use Severe Thunderstorm Warnings) for the perceived notion that QLCS tornadoes are weak (EF0 and EF1). Makes for excellent reading!
Greg Stumpf, CIMMS and NWS/MDL