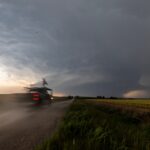
LIFT (Low-Level Internal Flows in Tornadoes) seeks to better understand winds in the lowest levels of tornadoes. To do so, scientists will have to get up close and personal.
LIFT (Low-Level Internal Flows in Tornadoes) seeks to better understand winds in the lowest levels of tornadoes. To do so, scientists will have to get up close and personal.
SCIENCE IMPACT: NSSL’s Warn-on-Forecast System yields 75 lead time on Greenfield, Iowa tornado, demonstrating potential for long-range tornado warnings.
NSSL’s Low-Level Internal Flows in Tornadoes experiment, or “LIFT”, intercepted a violent tornado southeast of Duke, Okla., gathering a data set that could prove to be significant in our understanding of tornado winds at the ground level.
The Warn-on-Forecast System is a revolutionary approach to forecasting severe weather and tornadoes that could lead to a historic leap in warning lead times.
The NOAA National Severe Storms Laboratory is back in the field, testing tornado hypotheses with the DELTA Project.
The United States Department of Commerce announced on Tuesday that the Forecast Research and Development Division at NOAA’s National Severe Storms Laboratory (NSSL) is a recipient of its highest award, a Gold Medal.
For more than 60 years, NSSL researchers have been taking to the field to study tornadoes and severe weather.
The NOAA National Severe Storms Laboratory (NSSL) hosted its inaugural NSSL Science and Engineering Day recently, gathering lab staff for a full day of reflection, discussion and collaboration. With a focus on appreciating the history and achievements of the lab while also casting a vision for the future, the event gave lab staff a unique opportunity to share, collaborate and think about the future of NSSL.
As Tropical Storm Hilary made landfall in California and affected the southwestern United States, researchers at the NOAA National Severe Storms Laboratory (NSSL) stepped into action, using a cutting-edge tool to lend support to forecasters.
The NOAA Hazardous Weather Testbed provides a conceptual framework and a physical space to foster collaboration between research and operations to test, perfect and evaluate emerging technologies and science for NWS operations.