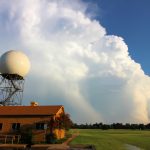
Downbursts—an area of strong winds in a thunderstorm—can damage trees and buildings, disrupt air travel, and cause loss of life. Decades of work by scientists has revealed a lot of information about downbursts including certain…
Downbursts—an area of strong winds in a thunderstorm—can damage trees and buildings, disrupt air travel, and cause loss of life. Decades of work by scientists has revealed a lot of information about downbursts including certain…
Weather models are the basic building blocks of a forecast. Researchers leverage machine learning techniques in an effort to improve these tools.
For the month of October NOAA National Severe Storms Laboratory is publishing a series of stories highlighting some of the women working at the lab. One Q&A segment will be published each Monday in October.…
Alexander Ryzhkov is a senior research scientist with over 20 years of experience at CIMMS/NSSL. Learn more.
A new program supported by NSSL is testing the use of stream radar to improve flood forecasting. Learn more.
Yunheng Wang is part of the Warn-on-Forecast team, developing software for atmospheric applications. Learn more.
Heather works with NSSL’s Warning Research Development Division, managing NSSL’s FAA research portfolio. Learn more.
Matt is part of the Severe Weather Warning Applications and Technology Transfer group in WRDD. Learn More.
John’s passion is in chaos theory and the predictability of weather. Learn what he does with CIMMS/NSSL!
Katie is a Ph.D. candidate at OU, studying the impact of rapid-scan radar data on forecaster warning decision-making. Learn more.