Weather models are the basic building blocks of any forecast. NOAA National Weather Service forecasters utilize a variety of models to provide accurate weather information for the public when severe weather threatens.
NOAA and cooperative institute researchers are leveraging machine learning techniques and high resolution weather models in an effort to improve these tools.
“We hope our research will provide forecasters with more information on when they should, or shouldn’t, rely on certain forecast models,” said Burkely Gallo, a researcher at the University of Oklahoma Cooperative Institute for Mesoscale Meteorological Studies, whose work supports the NOAA NWS Storm Prediction Center.
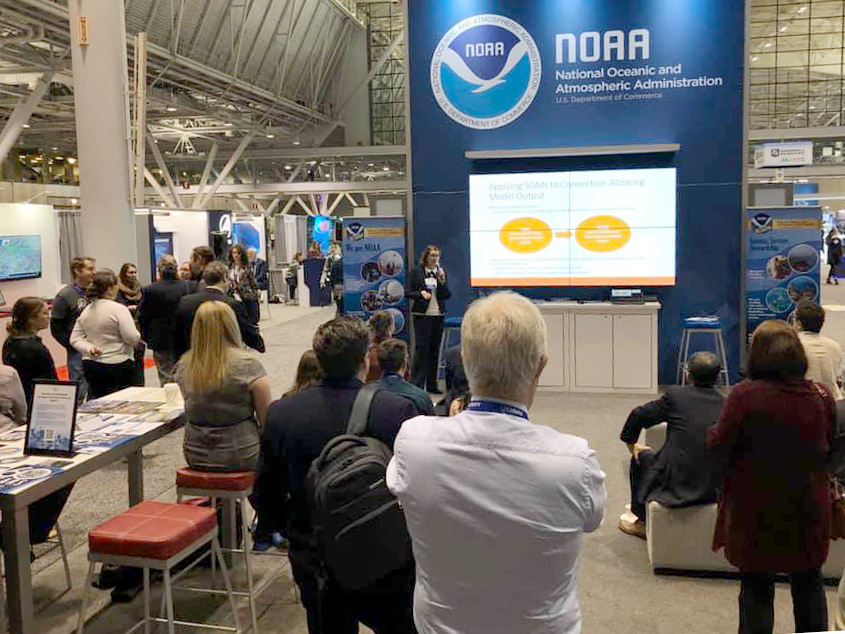
Computer weather models continue to improve, providing accurate forecasts as much as a week in advance. Yet, each has strengths and weaknesses and must be interpreted by knowledgeable human forecasters. It could take decades of experience for forecasters to gain expertise on what forecasting models are the most accurate for specific weather events.
A series of preliminary research aims to allow automatic flagging of problematic forecasts, provide quality control for the development of new atmospheric models and allow model developers to learn why a model is or is not valid.
“To achieve the latest and greatest forecasting models, people developing the models need to know how they are performing in certain scenarios,” Gallo said. “We hope this can help them identify priorities for future model development.”
Alex Anderson-Frey is a co-researcher on the project, which began as an internal funding proposal in a competition organized by the NOAA Central Regional Collaboration Team. Anderson-Frey and Gallo won funding for their project and their work was supported by NOAA’s National Severe Storms Laboratory when it began.
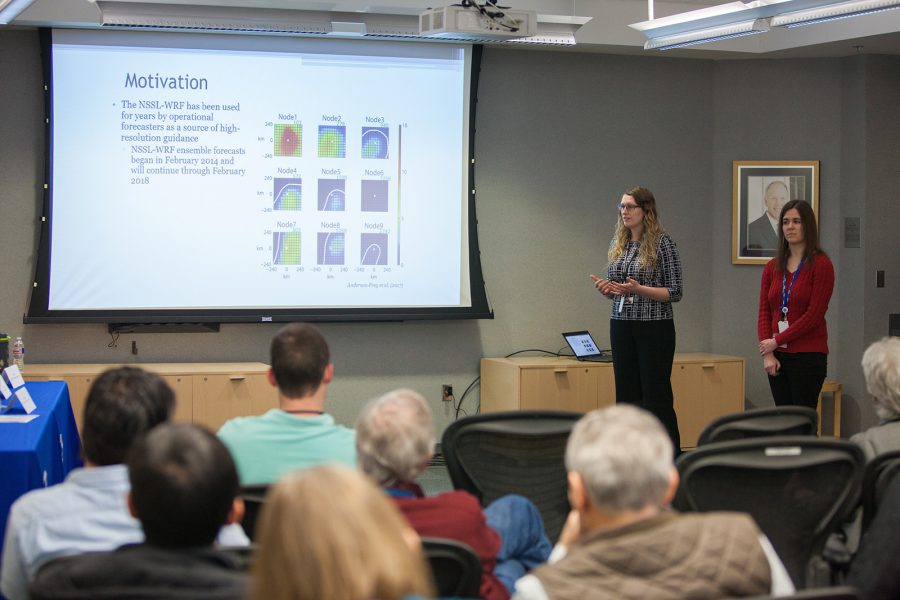
“Alex and I have wanted to work together since college,” Gallo said. “We decided this internal program could be the spark for collaboration.”
Gallo and Anderson-Frey used the competition funding to hire a graduate student part-time and the result of his efforts allowed them to have a complete dataset to begin their work, which leverages machine learning. Machine learning sorts storms based on different environmental factors surrounding the storms. Environmental factors include fields, like dew point and temperature. Found patterns can then be matched to a current model forecast. This provides forecasters an idea of how the model they are using performed in similar past scenarios.
“We want to provide tools that allow forecasters to quickly learn, so they can know if a model has statistically performed very well for tornado detection in this type of model environment,” Gallo said. “Forecasters manage a fire hose of data and we hope to make the fire hose manageable.”
Gallo said she expects the project to continue for several years, with the team’s goal of testing the products in NOAA’s Hazardous Weather Testbed.