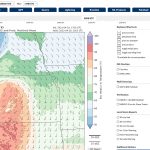
The Warn-on-Forecast System is a research project at the NOAA National Severe Storms Laboratory that aims to increase lead time for tornado, severe thunderstorm and flash flood warnings. Since its inception in 2009, researchers have…
The Warn-on-Forecast System is a research project at the NOAA National Severe Storms Laboratory that aims to increase lead time for tornado, severe thunderstorm and flash flood warnings. Since its inception in 2009, researchers have…
Downbursts—an area of strong winds in a thunderstorm—can damage trees and buildings, disrupt air travel, and cause loss of life. Decades of work by scientists has revealed a lot of information about downbursts including certain…
The first week of April concluded the 2021 Warn-on-Forecast Testbed Experiment as part of the NOAA Hazardous Weather Testbed. Like many scientific activities, this experiment was delayed and then moved virtually due to the ongoing COVID-19 pandemic.
The Annual Meeting of the American Meteorological Society is the largest recurring conference in our field. In January 2021, the 101st AMS took place virtually, but that didn’t stop the experimental Warn-on-Forecast System from taking center stage.