I went ahead and used some educated guesses to develop model proxies for severe storms in the model. But how do those modeled reports compare to observed reports? This question, at least the way it is addressed here, yields an interesting result. Lets go to the figures:

The 2 images show the barchart of all the dates on the left, with the Modeled reports (top), observed reports close to modeled storms (middle) and the natural log of the pixels of each storm (or area; bottom) on the right. The 1st image has the modeled storm reports selected and it should be pretty obvious I have chosen unwisely (either the variable or the value) for my hail proxy (the reports with a 2 in the string). Interestingly, the area is skewed to the right or very large objects tend to be associated with model storms.
Also note that modeled severe storms are largest in the ensemble for 24 May with 27 Apr coming in 6th. 24 May appears first in percent of storms on that date with the 27 Apr outbreak coming in 15th place (i.e. having a lot of storms that are not severe).
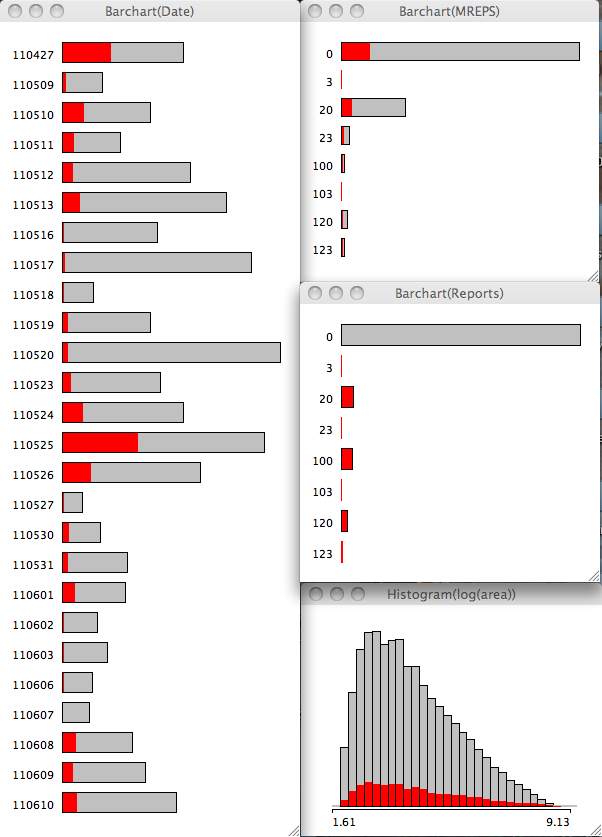
Changing our perspective and highlighting the observed reports that are close to modeled storms, the storm area distribution switches to the left or smallest storm area.
The modeled storms to verify has 25 May followed by 27 Apr coming in with the most observed reports close by. 24 May lags behind in 5th place. In a relative sense, 27 Apr and 25 May switch places, with 24 May coming in 9th place.
These unique perspectives highlight two subtle but interesting points:
1. Modeled severe storms are more typically larger (i.e. well resolved),
2. Observed reports are more typically associated with smaller storms.
I believe there are a few factors at play here including the volume and spacing of reports on any particular day, and of course how well the model performs. 25 May and 27 Apr had lots of reports so they stand out. Plus all the issues associated with reports in general (timing and location uncertainty). But I think one thing also at work here is that these models have difficulty maintaining storms in the warm sector and tend to produce small, short-lived storms. This is relatively bad news for skill; but perhaps a decent clue for forecasters. I say clue because we really need a larger sample across a lot of different convective modes to make any firm conclusions.
I should address the hail issue noted above. I arbitrarily selected an integrated hail mixing ratio of 30 as the proxy for severe. I chose this value after checking out the 3 severe variable (hourly max UH > 100 m s-2 for tornadoes, hourly max wind > 25.7 m s-1, hourly max hail > 30) distributions. After highlighting UH at various thresholds it became pretty clear that hail and UH were correlated. So I think we need to look for a better variable so we can relate hail-fall to modeled variables.