*Authored by Researchers Jooho Kim, Patrick Campbell, and Communications Specialist Emily Jeffries. For more information on this project, or to collaborate with the researchers involved, please email nssl.outreach@noaa.gov*
Severe weather hazards such as hail, high wind speeds, and tornadoes, can impact essential community infrastructure. Researchers are studying the impacts of severe weather threats on a range of community assets, including critical infrastructures like hospitals, fire stations, and schools, to improve the resiliency of communities.
Researchers from the Cooperative Institute for Mesoscale Meteorological Studies at the University of Oklahoma, in collaboration with the NOAA National Severe Storms Laboratory, say these studies could support National Weather Service forecasters, emergency managers, and the public by providing advance notice of the physical risks from severe weather threats.
Researchers recognized a need to effectively help communities predict damage to buildings and other physical impacts of severe weather threats. Prediction of such damage could improve communities’ abilities to manage their preparedness, response, and recovery phases for emergency or disaster management.
Outcomes of this research study may also enhance existing operation systems by providing real-time damage estimates for critical infrastructure and building properties.
The Tools and Results
For an effective community risk assessment from severe weather threats, two components are crucial — accurate weather information and a geodatabase of community assets. This research utilized experimental Probabilistic Hazard Information (PHI) as a weather information source.

In recent years, a prototype software system that allows forecasters to generate PHI was developed under the Forecasting A Continuum of Environmental Threats (FACETs) program at NSSL and CIMMS.
PHI for severe weather threats can be represented by continuously updating probabilistic hazard grids, which map the likelihood of an hazard occurring. PHI can be tailored and adapted to meet a variety of needs to effectively predict and communicate the risk of hazardous weather to forecasters, emergency agencies, and communities.
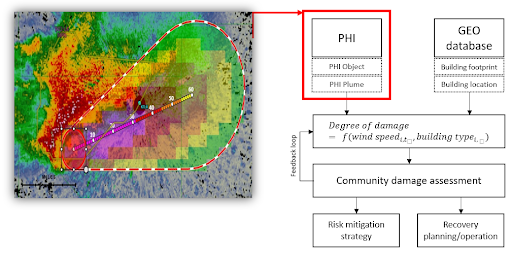
In order to leverage PHI in the assessment of community risk, researchers recognized a need to develop a geodatabase — a database designed to store and query geographic information — for community assets. This information includes multiple building types, like residential, commercial, and industrial, and critical infrastructure.
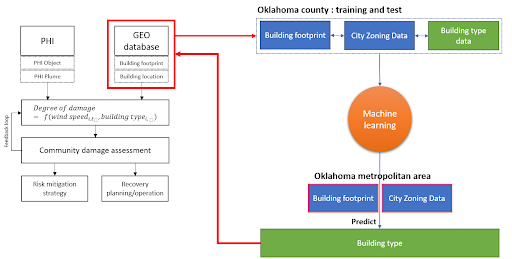
Using the Enhanced Fujita Scale (EF-Scale), researchers are testing the possibility of estimating the Degree of Damage (DoD) to individual buildings. The EF-Scale is the same standard used by the National Weather Service to rate tornado damage.
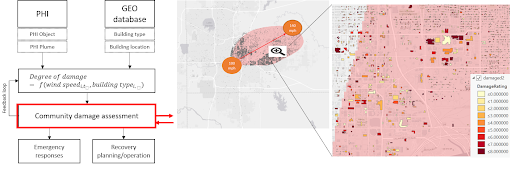
Researchers can successfully compute DoD given that a Damage Indicator (DI) and wind speed range are provided for buildings. Currently, the EF-Scale has 28 DIs corresponding to a wide range of building types, such as one- or two-family residences, manufactured homes, and apartments.
While building footprint data, building location, area, perimeter, and sometimes height is often provided by state and city offices, it can take tremendous time to manually categorize millions of different buildings into the 28 types of DI.
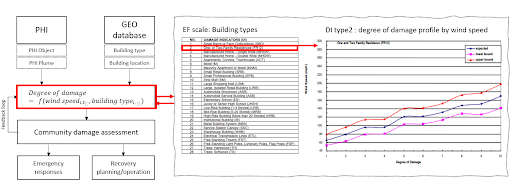
In order to identify DI types for large areas, researchers are using multiple cutting-edge machine-learning algorithms, making use of building footprint, city zoning ordinance data, images, and other publicly available data. As a geodatabase of DI information is built, researchers will be able to combine it with PHI data to produce detailed estimates of expected building damage and the likelihood of their occurrence.
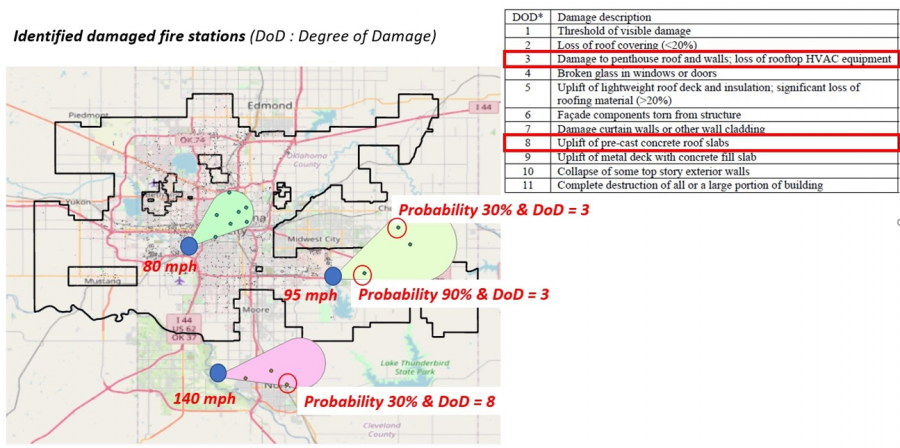
Potential Impact
If advance warning of damage to structures could be fully developed and incorporated into NWS operations, researchers expect it could become a valuable part of the comprehensive severe weather hazard information that is envisioned by the FACETs program.
Emergency managers could use information about at-risk community assets, including critical infrastructure, to maximize their mitigation and response efforts, and television broadcasters could use estimated damage information to focus their message. This enhanced hazard information can be used by the general public to make better decisions to protect themselves when under threat from severe weather.